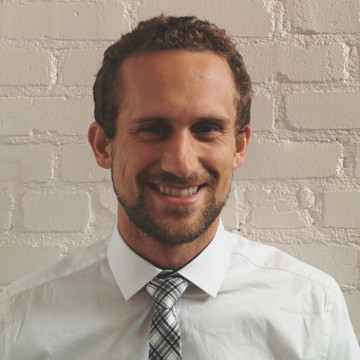
Andrew D. White
Associate Professor of Chemical Engineering
PhD, University of Washington, 2013
- Office Location
- 4401 Wegmans Hall
- Telephone
- (585) 276-7395
- Fax
- (585) 273-1348
- Web Address
- Website
Research Overview
Andrew White's laboratory is focused on automating the process of doing science—including literature research, hypothesis generation, experiment planning, analysis. This encompasses building large language models that can reason over literature, capturing human preferences of literature, and building complex scientific agents that can interact with chemical and biological computational methods. Our papers have set records in automating literature research, performing molecular cloning, and we demonstrated the first closed-loop AI system that could do arbitrary chemistry tasks from natural language.
Research Interests
- Large language models
- Deep learning in chemistry
- Molecular dynamics
Courses Taught
- CHE 478: Machine Learning of Molecules and Materials
Selected Publications
- Complete Publication List
- White, A.D., "The future of chemistry is language," Nature Reviews Chemistry, 2023. DOI: 10.1038/s41570-023-00502-0
- Ansari, M,; White, A.D., "Serverless Prediction of Peptide Properties with Recurrent Neural Networks," Journal of Chemical Information & Modeling, 2023, 63, 8, 2546-2553. DOI: 10.1021/acs.jcim.2c01317
- Wellawatte, G.P.; Gandhi, H.A.; Seshadri, A.; White, A.D., "A Perspective on Explanations of Molecular Prediction Models," Journal of Chemical Theory and Computation, 2023, DOI: 10.1021/acs.jctc.2c01235.
- Ansari, M.; Soriano-Panos, D.; Ghoshal, G.; White, A.D., "Inferring spatial source of disease outbreaks using maximum entropy," Physical Review E, 2022, 106, 1. DOI: 10.1103/PhysRevE.106.014306
- Zhu, W.; Luo, J.; White, A.D., "Federated learning of molecular properties with graph neural networks in a heterogeneous setting," Patterns, 2022, 3, 6. DOI: 10.1016/j.patter.2022.100521
- Cox, S.; White, A.D., "Symmetric Molecular Dynamics," Journal of Chemical Theory & Computation, 2022. DOI: 10.1021/acs.jctc.2c00401
- Barrett, R.; Ansari, M.; Ghoshal, G.; White, A.D., "Simulation-based inference with approximately correct parameters via maximum entropy," Machine Learning-Science & Technology,2022, 3, 2, 025006. DOI: 10.1088/2632-2153/ac6286
- Ansari, M.; Gandhi, H.A.; Foster, D.G.; White, A.D., "Iterative Symbolic Regression for Learning Transport Equations," AIChE Journal, 2022, e17695. DOI 10.1002/aic.17695
- Wellawatte, G.P.; Seshadri, A.; White, A.D., "Model Agnostic Generation of Counterfactual Explanations for Molecules," Chemical Science, 2022, Early Access. DOI 10.1039/d1sc05259d
- Hamsici, S.; White, A.D.; Acar, H., "Peptide Framework for Screening the Effects of Amino Acids on Assembly," Science Advances, 2022, 8, 3, eabj0305. DOI: 10.1126/sciadv.abj0305
- Zhu, W.; White, A.D.; Luo, J., "Federated Learning of Molecular Properties in a Heterogeneous Setting," arXiv preprint, 2021, arXiv:2109.07258
- Hocky, G.M.; White, A.D., "Natural language processing models that automate programming will transform chemistry research and teaching," arXiv preprint, 2021, arXiv:2108.13360
- Ansari, M.; Gandhi, H.A..; Foster, D.G.; White, A.D., "Iterative symbolic regression for learning transport equations," arXiv preprint , 2021, arXiv:2108.03293
- Wellawatte, G.P.; Seshadri, A.; White, A.D.,"Model agnostic generation of counterfactual explanations for molecules," 2021. doi.org/10.33774/chemrxiv-2021-4qkg8
- Gandhi, H.A.; White, A.D., "City-wide modeling of vehicleto-grid economics to understand effects of battery performance," arXiv preprint, 2021, arXiv:2108.05837
- Yang, Z.; Chakraborty, M.; White, A.D., "Predicting Chemical Shifts with Graph Neural Networks," Chemical Science, 2021, Early Release. DOI 10.1039/d1sc01895g
- Barrett, R.; White, A.D., "Investigating Active Learning and Meta-Learning for Iterative Peptide Design," Journal of Chemical Information & Modeling,2021, 61, 1, 95-105. DOI/abs/10.1021/acs.jcim.0c009461
- Gandhi, H.A.; Jakymiw, S.; Barrett, R.; Mahaseth, H.; White, A.D., "Real-Time Interactive Simulation and Visualization of Organic Molecules," Journal of Chemical Education. 2020, 97, 11, 4189-4195. DOI/abs/10.1021/acs.jchemed.9b01161
- Amirkulova, D.B.; Chakraborty, M.;White, A.D., "Experimentally Consistent Simulation of A beta(21-30) Peptides with a Minimal NMR Bias, Journal of Physical Chemistry B, 2020, 124, 38, 8266-8277. DOI/abs/10.1021/acs.jpcb.0c07129
- Li, Z.; Wellawatte, G.P.; Chakraborty, M.; Gandhi, H.A.; Xu, C.; White, A.D., "Graph Neural Network Based Coarse-Grained Mapping Prediction," Chemical Science, 2020, 11, 35, 9524-9531. DOI: 10.1039/d0sc02458a
- Chakraborty, M.; Xu, J.; White, A.D., " Is Preservation of Symmetry Necessary for Coarse-Graining?" Physical Chemistry Chemical Physics, 2020, 22, 26, 14998-15005. DOI.org/10.1039/D0CP02309D
- Chakraborty, M; Ziatdinov, M.; Dyck, O.; Jesse, S. White, A.D.; Kalinin, S.V., "Reconstruction of the Interatomic Forces from Dynamic Scanning Transmission Electron Microscopy Data," Journal Applied Physics, 2020, 127, 22, 224301. DOI:10.1063/5.0009413
- Amirkulova, D.B.; White, A.D., "Recent Advances in Maximum Entropy Biasing Techniques for Molecular Dynamics," Molecular Simulation, 2019, 45, 14-15. DOI:10.1080/08927022.2019.1608988
- Barrett, R.; Jiang, S.; White, A.D., "Classifying Antimocrobial and Multifunctional Peptides with Bayesina Network Models," Peptide Science, 2018, 110, 4, e24079. DOI:10.1002/pep2.24079
- Mayer, H.B.; Lee, S.; White, A.D.; Voth, G.A.; Swanson, J.M.J., "Multiscale Kinetic Modeling Reveals an Ensemble of Cl-/H+ Exchange Pathways in ClC-ec1 Antiporter," Journal of the American Chemical Society,2018, 140, 5, 1793-1804. DOI/10.1021/jacs.7b11463
- Amirkulova, D.B.; White, A.D., "Combining Enhanced Samples with Experiment-Directed Simulation of the GYG Peptide," Journal of Theoretical & Computational Chemistry, 2018, 17, 03, 1840007. DOI.org/10.1142/S0219633618400072
- Barrett, R.; Gandhi, H.A.; Naganathan, A.; Daniels, D.; Zhang, Y.; Onwunaka, C.; Luehmann, A.; White. A.D., "Social and Tactile Mixed Reality Increase Student Engagement in Undergraduate Lab Activities," J. Chem. Educ., 2018, 10, 1021. DOI/10.1021/acs.jchemed.8b00212
- Chakraborty, M.; Xu, C.; White, A.D., "Encoding and Selecting Coarse-Grain Mapping Operators with Hierarchical Graphics," Chemical Physics, 2018, 149, 13, 10.1063.https://arxiv.org/abs/1804.04997v1
Honors
- Outstanding Early Career Researcher Award, Digital Discvery RSC, 2024
- Gleasen Young Eng. of the Year, Rochester Eng. Soc., 2021
- Outstanding Investigator Award (R35, MIRA), NIH, 2020
- Young Investigator Award, AIChE COMSEF 2020
- Institute for Pure & Applied Mathematics Senior Fellow, 2019
- Curtis Award for Nontenured Faculty Teaching, 2019
- NSF Career Award, 2018